
Data Mesh is a new way to organize and manage data that helps organizations overcome challenges with traditional data architectures. Zhamak Dehghani introduced the Data Mesh concept in 2019, and it has exploded in popularity due to its promise to help organizations to break down data silos, improve data quality, increase agility, and scale more effectively.
“Data Mesh is a decentralized sociotechnical approach to share, access, and manage analytical data in complex and large-scale environments—within or across organizations.”
– Zhamak Dehghani, Data Mesh
Data Mesh is comprised of four key principles:
Data-as-a-Product Thinking: Oftentimes, data professionals spend most of their time trying to find, collect, understand, and clean data—rather than spending their time using data. Data-as-a-Product represents organizational intentionality in serving useful data (called "Data Products") to data consumers, and shifting the responsibilities to make the data discoverable, useful, trusted, and understood to data producers (called “Data Product Owners”). Like traditional products, Data Products should be useful, easy to understand, and meet customers' requirements. Data consumers should not use the organization's source system data and should instead use Data Products to support their use cases.
By creating and serving Data Products to data consumers, organizations can ensure the right data is being used, for the right use cases, through data assets that are trustworthy and reusable. Data consumers can spend more of their time using data and can trust the data is of high quality.
Domain-driven Data Ownership: Large enterprises have many different departments and organizations each with their own unique data; Data Mesh calls these “Domains”. Traditionally, these enterprises rely on centralized data teams to be responsible for managing all data across the enterprise. However, this leads to inefficiencies, bottlenecks, and data silos.
Instead of having centralized data teams work across all Domains, each Domain has its own self-sufficient data team, led by a Data Product Owner, that creates, serves, and improves data products for that Domain. These Data Product Owners are accountable for delivering their Domain’s Data Products to the mesh, determining access requirements, maintaining documentation, and intaking requirements from the enterprise for creating new Data Products or improving existing Data Products.
There are three Domain types within a Data Mesh:
Source-aligned Data Domains: These Domains work directly with raw source system data to create Data Products that are cleansed, curated, and useful for other Domains. These Data Products will resemble the source system data while being much easier to understand and work with for other Domains than working with the raw data itself.
Aggregate-aligned Data Domains: These Domains integrate Data Products to create new Data Products that represent higher-order, canonical business entities. These are sometimes called "360 views" of a standard business concept or object and typically contain a list of attributes about the object and a defined set of relationships to other objects.
Purpose-aligned Data Domains: These Domains integrate Data Products to create new Data Products that serve a specific business need. These Domains typically create Data Products that support decision-making. By contrast, the other Domains typically create Data Products used for reporting and analytics and get consumed by Purpose-aligned Domains.
Self-service Infrastructure: To operate as autonomous Domains effectively, these Data Product Owners and consumers must be self-sufficient. There are countless approaches for technical implementation on how to accomplish this, but it must make the process to discover, access, use, govern, and share Data Products seamless. In many cases, an organization can shift towards a Data Mesh through the tools and platforms it already has in place.
If using many different technologies and platforms across the mesh, interoperability, protocol standardization, and data-technology decoupling are critical to ensure Data Products are created and shared seamlessly. If using a monolithic technology stack, access controls, scalability, and flexibility are critically important to meet the unique needs of each Data Domain across the enterprise.
Federated Computational Governance: If done improperly, shifting towards decentralized and autonomous Data Domains could devolve into disorderly and anarchic communities that create high-quality data silos. Certain considerations must remain centralized at the enterprise level to ensure an orderly and federated operation. For example, organizational data standards, policies, and incentivization should be centralized while pushing data quality, documentation, and security down to the Domain level. Additionally, for large organizations, Data Mesh services must be well-defined and robust at the enterprise-level to ensure data product interoperability across the enterprise.
The process of governing data is often time-consuming and people-intensive, especially when the data is sensitive. Federated Computational Governance mandates using automation as much as practical to accomplish the federated governance approach outlined above.
Conclusion
Shifting towards a Data Mesh implementation is as much, if not more, of a people and process transformation as a technology transformation. An organization cannot buy a Data Mesh, and implementation will only be successful with that organization driving the transformation required to operate a Data Mesh.
Industry is still exploring Data Mesh and there is not a singular approach to implementation. Different organizations are successfully implementing a Data Mesh in different ways, based on the unique needs of their enterprise.
Get in touch to find out how LMI can support Data Mesh exploration and implementation for your organization.
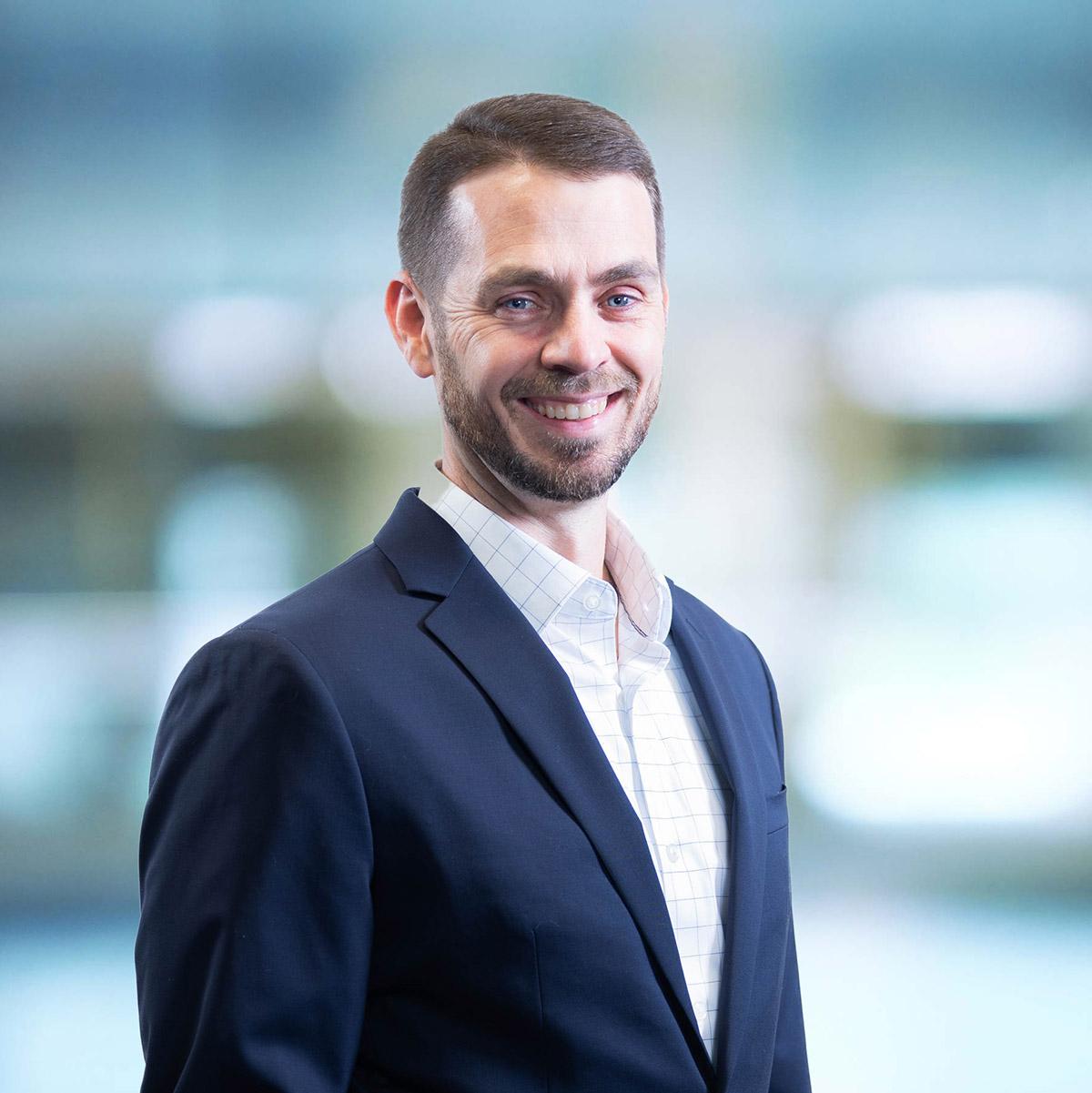